Deep Learning Indaba 2024 highlights: Strengthening AI in Africa
Learn about the key themes in machine learning presented at the Indaba 2024 including data-centric AI, the need for robust models and better data management.
Deep Learning Indaba 2024 Highlights: Strengthening AI in Africa
We are excited to share highlights from the Deep Learning Indaba 2024, a landmark event held 10 weeks ago in Dakar, Senegal. With its mission to strengthen machine learning (ML) and artificial intelligence (AI) research and development across Africa, the event brought together leading researchers, practitioners, and industry leaders to discuss key challenges and breakthroughs in AI.
‍
Event Overview
The Deep Learning Indaba 2024 showcased the latest advancements in AI research and applications, supported by an impressive roster of leading AI companies. Leaders in the field such as OpenAI, Apple, Google DeepMind, Meta, NVIDIA, and Microsoft AI for Good Lab, among others, lent their support to this prestigious gathering.
Amidst this gathering, Dotphoton was honored to contribute to the event. Our Head of AI/ML, Luis Oala, presented an insightful talk on paradoxes in data-centric machine learning, highlighting challenges and new approaches that are essential for future-proofing AI models.
‍
Key Highlights from Luis Oala’s Talk
‍
Data-Centric Machine Learning: A Paradigm Shift
Luis began by introducing the Data-centric Machine Learning Research (DMLR) community, an impressive collaboration of nearly 40 scientists from organizations such as Dotphoton, NASA, Google, TU Delft, Carnegie Mellon University, UC Berkeley, and others.
This shift towards data-centric ML represents a fundamental change in how we approach AI development. For those interested in delving deeper into this topic, Luis recommended the comprehensive paper “DMLR: Data-centric Machine Learning Research - Past, Present, and Future” (https://arxiv.org/abs/2311.13028).
‍
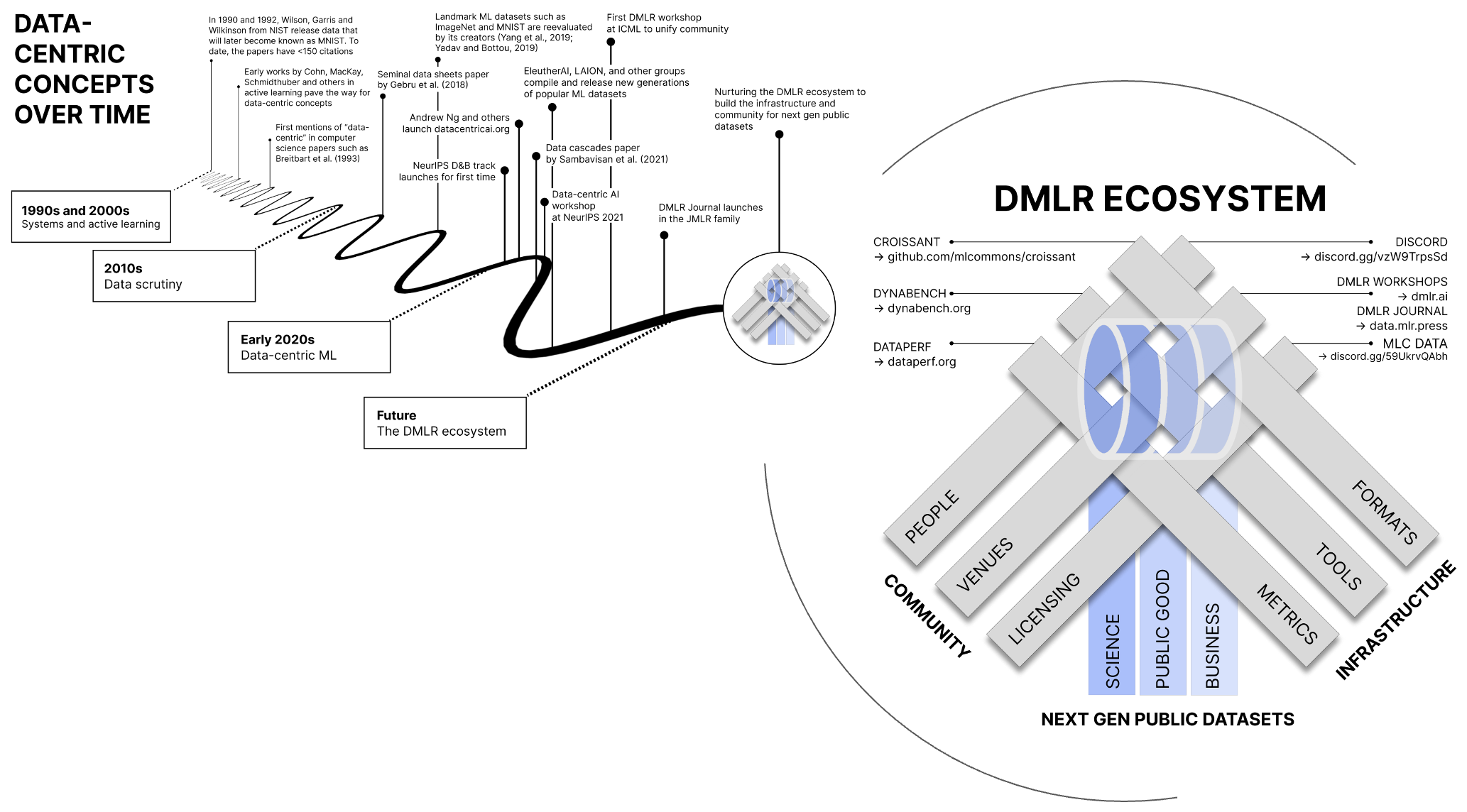
‍
The Data Dilemma: Balancing Scale and Quality
Building on the data-centric theme, Luis explored one of the central paradoxes in modern machine learning: the tension between the need for large datasets and the necessity of maintaining high data quality. This dilemma lies at the heart of data-centric ML, where the focus has shifted from model architecture to data management and quality.
‍
As datasets grow ever larger, ensuring their quality becomes increasingly challenging yet crucial. Luis emphasized that this shift from model-centric to data-centric approaches is not just a trend, but a necessary evolution in the field of AI. It requires us to develop new methodologies and tools to manage, clean, and validate data at scale.
‍
Navigating the Challenges of Semi-Supervised Deep Learning
Transitioning from the broader data quality discussion, Luis delved into the specific challenges faced in semi-supervised deep learning (SSDL). He highlighted a significant issue in this domain: distribution mismatch, which occurs when the labeled and unlabeled datasets have differing data distributions.
‍
This mismatch can lead to poor generalization, as the model learns from labeled data that doesn’t accurately represent the unlabeled data. To address this issue, Luis discussed research on dataset similarity metrics, referencing the work by Calderon-Ramirez, S., Oala, L., et al. (2022) titled “Dataset Similarity to Assess Semisupervised Learning Under Distribution Mismatch Between the Labeled and Unlabeled Datasets” (IEEE Transactions on Artificial Intelligence, 42, 282-291).
‍
These metrics aim to better align the labeled and unlabeled datasets, potentially improving model performance and reliability in SSDL tasks. This approach represents a step towards more robust and adaptable AI systems that can handle real-world data complexities.

‍
Taming the Drift: Dataset Drift Control
Building on the theme of data challenges, Luis emphasized the critical importance of detecting and addressing dataset drift. This phenomenon, where the distribution of incoming data changes over time and deviates from the original training data, is a recurring challenge in real-world ML applications.
To illustrate the importance of proactive dataset drift control, Luis referenced work by Dotphoton and academic partners, specifically in the context of optical images. He highlighted the paper by Oala, L., Aversa, M., et al. (2023) titled “Data Models for Dataset Drift Controls in Machine Learning With Optical Images” (Transactions on Machine Learning Research).
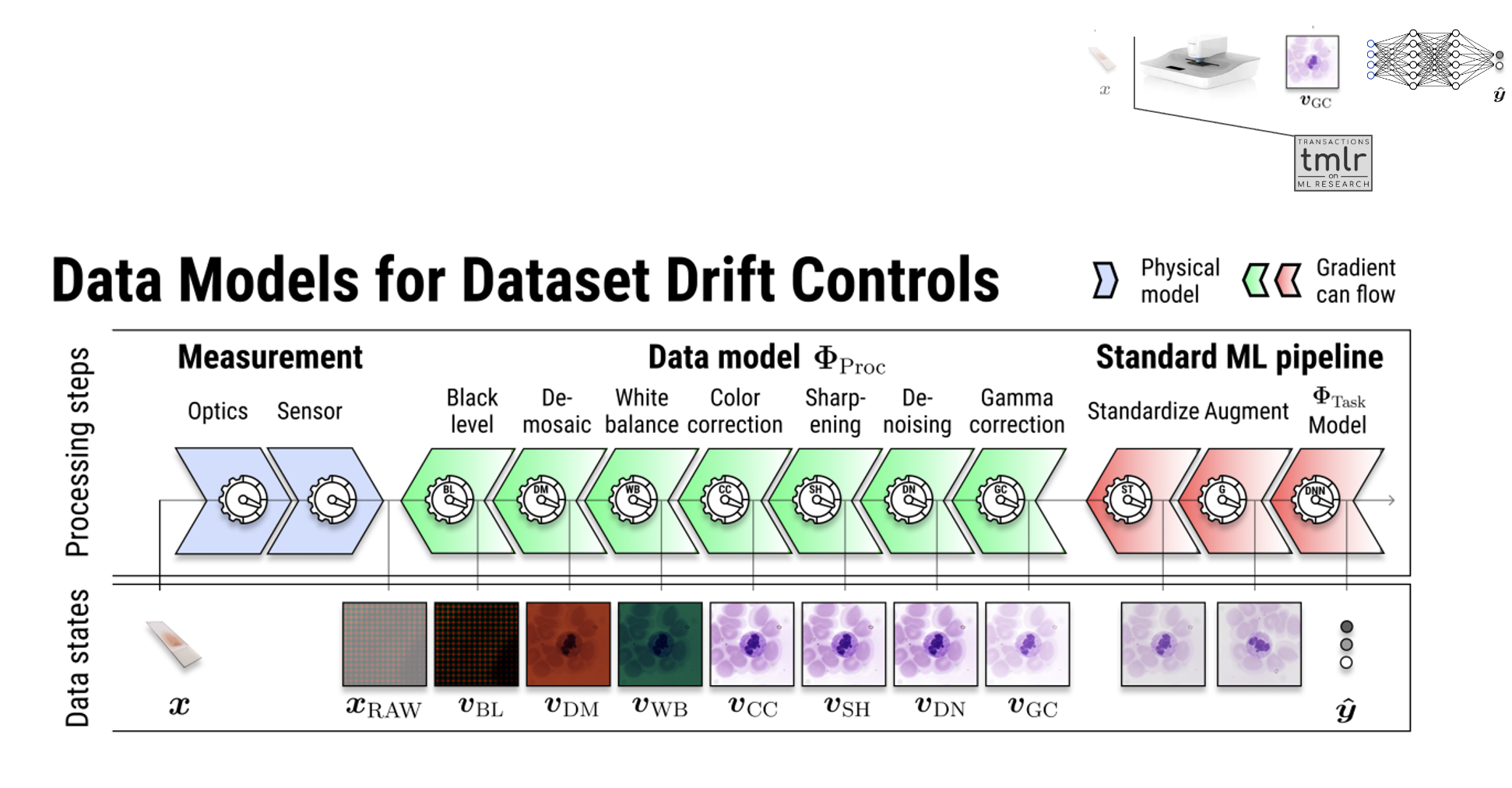
‍
This research discusses methodologies for implementing data models that monitor and adjust for drift, ensuring that models remain robust and accurate even as data evolves over time. Such approaches are crucial for maintaining the long-term reliability and performance of AI systems in dynamic, real-world environments.
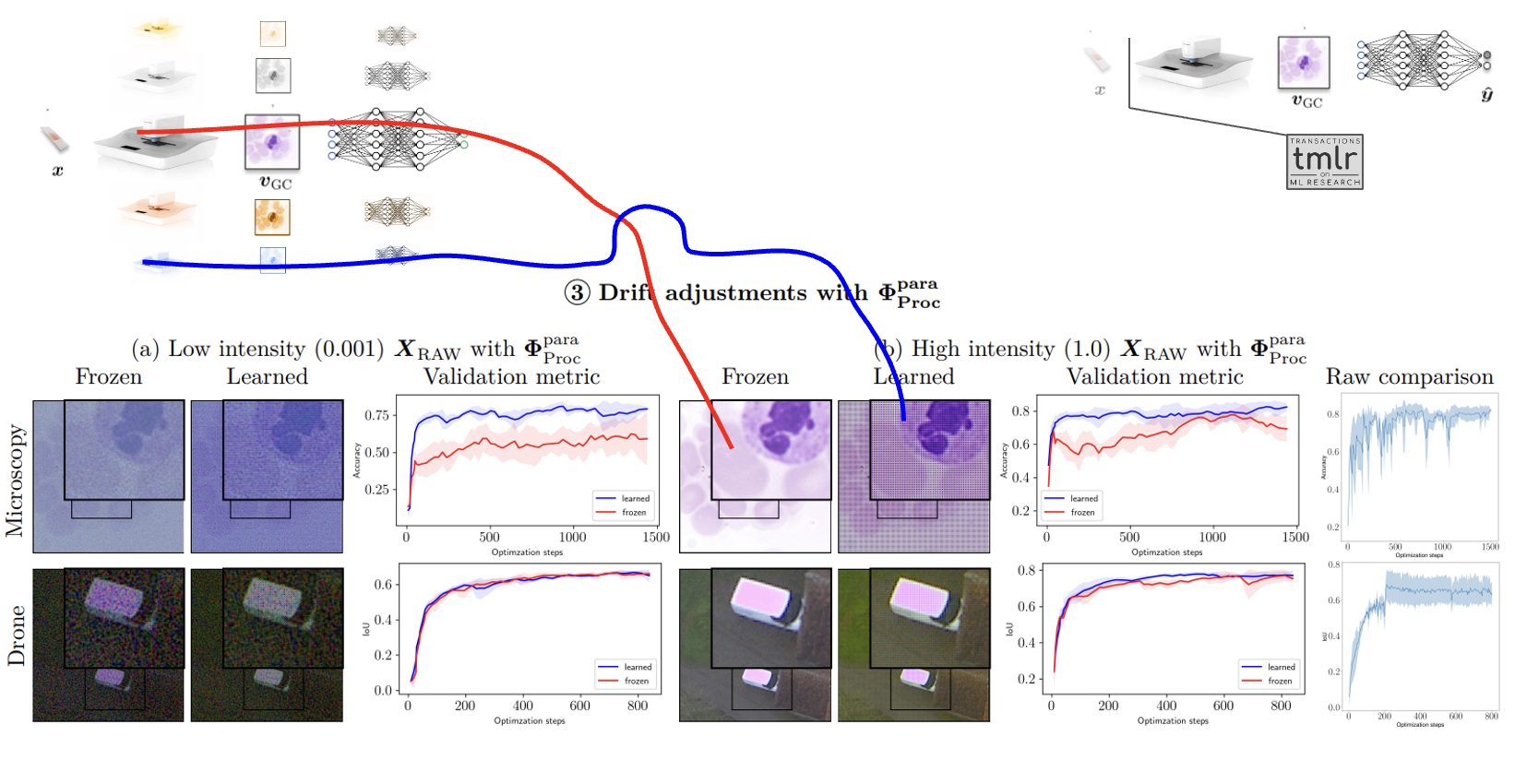
‍
The Power of Synthesis: Synthetic Data Generation
As the discussion moved from data challenges to solutions, Luis highlighted the crucial role of synthetic data in overcoming data scarcity. He emphasized its ability to simulate edge cases and unseen conditions, thereby helping models generalize more effectively.
‍
Luis referenced recent work exploring the use of large language models (LLMs) for synthetic data generation, curation, and evaluation, such as the comprehensive survey by Long, L., Wang, R., et al. (2024) titled “On llms-driven synthetic data generation, curation, and evaluation: A survey” (arXiv preprint arXiv:2406.15126).
He also presented DiffInfinite, a project he co-authored with Dotphoton and academic colleagues. This innovative approach generates high-quality synthetic images for histopathology through parallel random patch diffusion (Aversa et al., 2023, “DiffInfinite: Large Mask-Image Synthesis via Parallel Random Patch Diffusion in Histopathology”, Conference on Neural Information Processing Systems Datasets and Benchmarks Track).
Luis stressed that while synthetic data offers immense potential, it is important to consider curating and filtering it for utility. He referenced the work of Alaa et al. (2022), “How faithful is your synthetic data? sample-level metrics for evaluating and auditing generative models” (International Conference on Machine Learning, pp. 290-306. PMLR), which developed sample-level metrics for evaluating and auditing the reliability of generative models.
‍
Embracing Complexity: A Holistic Approach to Machine Learning
Drawing an insightful parallel, Luis compared the challenges in machine learning to the ancient parable of the blind men and the elephant. Just as each blind man perceives only a part of the elephant, leading to conflicting conclusions, ML practitioners often tackle different facets of machine learning—such as compression, control, generalization, invariance, and robustness—in isolation.
This analogy served to emphasize the importance of a holistic approach to machine learning. Luis argued that just as understanding the full elephant requires piecing together all parts, advancing AI requires integrating various aspects of machine learning to create more robust and versatile models.
Building the Future:
‍
Composable Infrastructure

As the talk neared its conclusion, Luis highlighted the growing prioritization of data preparation, validation, and transformation in the AI community. He introduced Croissant, a new metadata format designed to standardize machine learning datasets, as an example of the tools being developed to support this shift.
Developed by scientists from Dotphoton, Bayer, Meta, Google, NASA, and others, Croissant aims to ensure datasets are interoperable across platforms, enhancing collaboration and improving data-centric ML workflows. By streamlining metadata handling, reducing errors, and boosting productivity for ML teams, tools like Croissant play a crucial role in improving the reliability and usability of machine learning models, ultimately leading to faster development and better outcomes.

‍
Conclusion
The Deep Learning Indaba 2024 stands as a testament to the vibrant and growing AI research community in Africa. By bringing together leading researchers, practitioners, and industry experts, the event fostered collaboration and knowledge sharing that will undoubtedly contribute to the advancement of AI on the continent and beyond.
The shift towards data-centric AI, presents both new opportunities and challenges. It paves the way for more robust, adaptable, and reliable AI systems, while also demanding new approaches to data management and quality control.
As we look to the future, events like the Deep Learning Indaba play a crucial role in shaping the direction of AI research and development. They provide a platform for sharing ideas, fostering collaborations, and inspiring the next generation of AI researchers and practitioners. The insights and connections made at this event will continue to ripple through the AI community, driving innovation and progress in the field for years to come.
‍
Notable Speakers and Tutorials
The event featured inspiring talks by notable speakers, each bringing unique insights and expertise to the conference:
- Samy Bengio (Apple)
- Judy Gichoya (Emory University)
- Caglar Gulcehre (Google DeepMind)
- Isabel Valera (Saarland University)
- Marwa Mahmoud (Cambridge University)
- Marcellin Atemkeng (Rhodes University)
- Avishkar Bhoopchand (Google DeepMind)
- Martha Yifiru Tachbelie (Addis Ababa University)
- Khalil Mrini (TikTok)
- Girmaw Abebe (Microsoft AI for Good)